
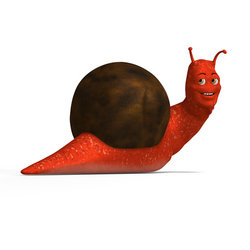
We provide more details about the architecture and training procedure in our paper. DALL♾ uses the standard causal mask for the text tokens, and sparse attention for the image tokens with either a row, column, or convolutional attention pattern, depending on the layer. The attention mask at each of its 64 self-attention layers allows each image token to attend to all text tokens. Finally, the transformations “a sketch of the animal” and “a cell phone case with the animal” explore the use of this capability for illustrations and product design.ĭALL♾ is a simple decoder-only transformer that receives both the text and the image as a single stream of 1280 tokens-256 for the text and 1024 for the image-and models all of them autoregressively. Those that only change the color of the animal, such as “animal colored pink,” are less reliable, but show that DALL♾ is sometimes capable of segmenting the animal from the background. Other transformations, such as “animal with sunglasses” and “animal wearing a bow tie,” require placing the accessory on the correct part of the animal’s body. This works less reliably, and for several of the photos, DALL♾ only generates plausible completions in one or two instances. The transformation “animal in extreme close-up view” requires DALL♾ to recognize the breed of the animal in the photo, and render it up close with the appropriate details. The most straightforward ones, such as “photo colored pink” and “photo reflected upside-down,” also tend to be the most reliable, although the photo is often not copied or reflected exactly. We find that DALL♾ is able to apply several kinds of image transformations to photos of animals, with varying degrees of reliability. We test DALL♾’s ability to modify several of an object’s attributes, as well as the number of times that it appears. The samples shown for each caption in the visuals are obtained by taking the top 32 of 512 after reranking with CLIP, but we do not use any manual cherry-picking, aside from the thumbnails and standalone images that appear outside. We illustrate this using a series of interactive visuals in the next section. We find that DALL♾ is able to create plausible images for a great variety of sentences that explore the compositional structure of language. In the future, we plan to analyze how models like DALL♾ relate to societal issues like economic impact on certain work processes and professions, the potential for bias in the model outputs, and the longer term ethical challenges implied by this technology. We recognize that work involving generative models has the potential for significant, broad societal impacts. This training procedure allows DALL♾ to not only generate an image from scratch, but also to regenerate any rectangular region of an existing image that extends to the bottom-right corner, in a way that is consistent with the text prompt. It receives both the text and the image as a single stream of data containing up to 1280 tokens, and is trained using maximum likelihood to generate all of the tokens, one after another. Like GPT-3, DALL♾ is a transformer language model. We extend these findings to show that manipulating visual concepts through language is now within reach. Image GPT showed that the same type of neural network can also be used to generate images with high fidelity.

GPT-3 showed that language can be used to instruct a large neural network to perform a variety of text generation tasks.
